太阳活动区EUV图像的生成式模型耀斑分级与预报 |
| |
引用本文: | 郭大蕾, 张振, 朱凌锋, 薛炳森. 太阳活动区EUV图像的生成式模型耀斑分级与预报[J]. 空间科学学报, 2023, 43(1): 60-67. doi: 10.11728/cjss2023.01.220214015 |
| |
作者姓名: | 郭大蕾 张振 朱凌锋 薛炳森 |
| |
作者单位: | 1.中国科学院自动化研究所 北京 100190;2.中国科学院大学人工智能学院 北京 100049;3.国家卫星气象中心 北京 100081 |
| |
基金项目: | 国家自然科学基金项目资助(11272333) |
| |
摘 要: | 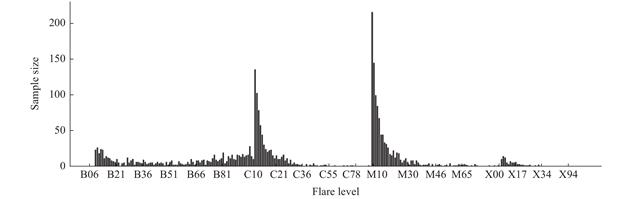 近年来,不断发射的空基观测台持续传送回海量日面图像及日地间气象数据,为采用人工智能技术对太阳活动进行预报预警提供了数据基础。但是,极端天气爆发少,样本量较少;中等程度爆发稍多,样本量较多;常规无爆发天气常见,样本较为集中,样本不均衡状况严重影响机器学习方法在空间天气领域的广泛应用。本文面向多源多通道多尺度日面图像信息,构建了来自SOHO和SDO的1996-2015年日面活动区图像数据集;针对数据分布的不平衡,对太阳活动区图像作耀斑分级与预报。在对比分析元学习算法的基础上,设计了结合分类头设计和卷积核初始化的生成式模型;在使网络轻量化的基础上,能够将M和X级耀斑预报的检测率指标相较于普通的深度学习模型和无监督度量式模型分别提升10%和7%。
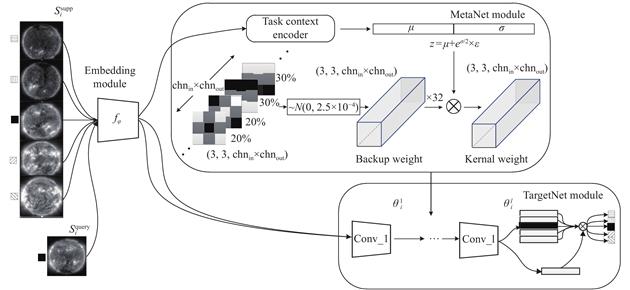
|
关 键 词: | 日面图像 耀斑 分级 生成式模型 人工智能 |
收稿时间: | 2022-02-13 |
修稿时间: | 2022-10-14 |
|
| 点击此处可从《空间科学学报》浏览原始摘要信息 |
|
点击此处可从《空间科学学报》下载全文 |
|